MIT Ph.D. proposes a verifiable controller framework, providing a solution for c
In recent years, neural network control technology has shown astonishing performance in the field of robotics. This technology, based on deep learning, has driven various advanced robots to complete a series of complex tasks.
For MIT doctoral student Yang Lujie, she can easily name these advanced robots.
Figure | Yang Lujie (Source: Yang Lujie)
For example:
There is the Figure general humanoid robot that can make coffee and actively engage in conversation with people; there is the ALOHA manipulator robot that can tie shoelaces and hang clothes; and there are also robot dogs that can cross various terrains to perform reconnaissance missions, etc.Behind all of these intelligent robots, there are carefully designed neural network controllers operating.
Although neural network control has shown excellent practicality, there is still a lack of stability assurance for safety-critical applications.
In the field of control theory, some scholars have provided rigorous stability proofs for linear controllers or polynomial controllers through norm optimization and formal methods.
However, there are still some challenges to provide the same stability assurance for more complex neural network controllers.
For neural network-based controllers, conducting rigorous Lyapunov stability verification is of great significance, mainly for the following reasons:
Advertisement
First, to ensure the safety and reliability of the system.Robots, autonomous vehicles, industrial control systems, and more all involve complex dynamic processes. If the control system becomes unstable, it may lead to serious safety accidents.
Through Lyapunov stability verification, the progressive stability of the system within a certain region can be formally proven, thereby ensuring that the system can operate safely and reliably.
Secondly, expand the application of neural networks in key areas.
Due to the lack of stability guarantees, the application of current neural network controllers in safety-critical fields is still greatly limited.
Once their stability can be strictly verified, neural networks can be widely used in fields such as robotics, aerospace, and life sciences, thus exerting strong control performance.Thirdly, to compensate for the theoretical shortcomings of neural networks.
Despite the outstanding performance of neural networks in practice, they inherently lack a solid theoretical foundation.
By integrating with Lyapunov stability theory, theoretical support can be injected into neural network controllers to make up for their deficiencies in interpretability and reliability.
Fourthly, to promote the integration of control theory and machine learning.
Neural networks and Lyapunov theory respectively represent the latest advancements in the two major fields of machine learning and control theory.Lyapunov verification for neural network controllers is a strong attempt to combine these two fields, and is bound to promote the cross-integration of the two fields, leading to new theoretical breakthroughs.
Secondly, to address the challenges of complex systems.
As the control objects become increasingly complex, the single classical control method is no longer able to fully meet the needs.
For neural network controllers, they have the ability to learn complex mappings. If their stability can also be guaranteed, it will provide a brand new solution for controlling complex nonlinear systems.
Based on this, Yang Lujie and his team propose a new framework, which uses methods such as rapid empirical counterargument and regularization technology, aiming to learn neural network controllers and Lyapunov stability proofs at the same time, in order to be applicable to complex nonlinear dynamic systems.The new formalization method proposed by Yang Lujie and others not only defines a larger stable area that has never been involved in existing research, but also improves the constraints for stable derivatives, focusing only on the range of stable areas that can be proven.
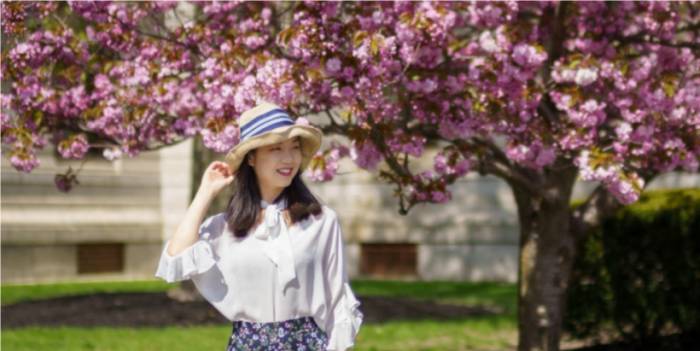
Through this novel approach, the research team has broken through the bottleneck of providing stability proofs for existing neural network controllers, providing a larger verifiable stable area for more complex neural network control systems.
For the verification of Lyapunov stability conditions, the team adopts a post-hoc rigorous verification method, using an expandable neural network verification technology based on linear boundary propagation, and employs branch and bound algorithms, as well as the current state-of-the-art α,β-CROWN neural network verifier, making the verification method both efficient and flexible.
The entire training process and verification process can be accelerated on the GPU (graphics processing unit, graphics processor), without relying on the expensive traditional solvers.
With this new type of verification, the use of expensive traditional solvers can be avoided, ensuring rigorous verification of Lyapunov stability conditions.At the same time, it also takes into account computational efficiency and flexibility, making the stability verification for neural network controllers more efficient and more feasible.
Through this, the research group has provided new solutions for the stable control of complex nonlinear systems, which have potential application prospects in the fields of industry, transportation, aerospace, and more.
Firstly, it can be used for robot control systems.
That is, the current method can be applied to the motion control of robots. Especially for robot systems with complex nonlinear dynamics, using the neural network controller and Lyapunov proof proposed in this study can ensure the stability and convergence of robot motion.
Secondly, it can be used for the control of autonomous driving and drones.Autonomous vehicles and drones often have nonlinear and high-dimensional dynamic equations.
By using the method presented in this paper, a neural network controller with guaranteed stability can be designed, which enhances the safety and reliability of autonomous driving and drones.
Thirdly, it can be used for the control of spacecraft and aircraft.
Spacecraft and aircraft typically have complex nonlinear dynamic models, and the method presented in this paper can provide a neural network control solution with guaranteed stability for them.
Fourthly, it can be used for the control of industrial processes.Many chemical engineering, metallurgical, and other industrial processes involve complex nonlinear dynamic systems. The method presented here can be used to design neural network controllers with guaranteed stability, optimizing the control performance of the production process.
Fifthly, it can be used for modeling and control of biomedical systems.
For some biomedical systems, such as gene regulatory networks, nonlinear dynamic models can be used to describe them. Therefore, the method presented here is expected to be applied to the modeling and control of these systems.
It is also reported that two weeks before the deadline for the relevant paper, the team re-examined the mathematical formulas in the paper.
In this discussion, they not only deeply optimized the formulas but also discovered errors and omissions in existing research in the field.Although the preliminary experimental results of this study have already far exceeded the most advanced achievements in the field at present. However, the above findings also led them to make a bold move: to overturn all existing experimental data and comprehensively design experiments based on the optimized formula.
"This is a challenging decision, because re-conducting experiments means great risks and additional workload, but we insist on ensuring the rigor and accuracy of the research results," said Yang Lu Jie.
After continuous fighting, they finally completed all experiments and the final revision of the paper 4 hours before the paper deadline, ensuring the high quality and innovation of this achievement.
Finally, the related paper was published in the International Conference on Machine Learning (ICML) with the title "Lyapunov-stable Neural Control for State and Output Feedback: A Novel Formulation for Efficient Synthesis and Verification" [1].
Yang Lu Jie and Dr. Dai Hongkai from the Toyota Research Institute are co-first authors.Next, the research team will explore the following areas.
Firstly, enhance the universality and adaptability of the model.
That is, develop methods based on neural networks to estimate or optimize the reliability of the system, as well as to tune and optimize other control indicators such as the value function and control barrier function, thereby improving the scalability and flexibility when dealing with complex dynamic systems.
Secondly, further integrate theory with practice.
Currently, the research team has made progress on drones, as well as on some standard control benchmarks.In the future, this framework can be applied to more and more complex control systems, such as industrial robots and autonomous vehicles, to verify and enhance their stability and reliability in complex practical scenarios.
Once again, interdisciplinary collaboration will be carried out.
Given the complexity and interdisciplinary nature of the research problem, they plan to collaborate with experts in the field of machine learning to strive for better solutions.
As previously mentioned, in order to synthesize a neural network controller with Lyapunov stability for general nonlinear dynamic systems, the research team also utilized the latest verification tools in the field of neural network verification, the α,β-CROWN ().
The α,β-CROWN was jointly developed by two collaborators of Yang Lujie - Professor Zhang Huan from the University of Illinois in the United States, and doctoral student Shi Zhouxing from the University of California.In the robustness verification of large-scale computer vision neural networks and the safety verification of neural network controllers, α,β-CROWN demonstrates outstanding scalability.
It leverages the network structure of the verification problem, accelerating computation by propagating linear boundaries within the neural network.
At the same time, the boundary propagation process of α,β-CROWN has good "GPU friendliness," supporting efficient verification of large networks, and can quickly evaluate multiple sub-problems with the help of branch and bound techniques.
Therefore, Yang Lujie also hopes to further utilize the capabilities of α,β-CROWN to solve more neural network control system verification problems.
Leave a Reply