The Shanghai Jiao Tong University team develops general artificial intelligence
"As a basic scientific researcher, the excitement I felt when I first saw our developed general artificial intelligence technology for protein engineering, which realized the design of protein sequences oriented towards functions and was successfully verified by wet experiments, was unparalleled," said Hong Liang, a distinguished professor at the School of Natural Sciences, School of Physics and Astronomy, and School of Pharmacy at Shanghai Jiao Tong University.
He further explained that this means that protein engineering, which previously relied on expert experience and a large number of experimental trials and errors, can now be designed directionally through general artificial intelligence, thereby reducing time and economic costs by several times or even dozens of times.
In addition, due to the universality of the model, it is applicable to various fields, which determines that it will greatly accelerate the development of China's biomanufacturing, synthetic biology, biomedicine, and other fields, helping Chinese enterprises to interact and compete with international leading companies in a healthy way.
The related paper, titled "Protein Engineering with Lightweight Graph Denoising Neural Networks," was published in the Journal of Chemical Information and Modeling [1].
Advertisement
Dr. Zhou Bingxin, a research assistant at the School of Natural Sciences at Shanghai Jiao Tong University, is the first author, and Professor Hong Liang serves as the corresponding author.Nowadays, the universal artificial intelligence for protein design, AccelProtein™, developed by Hong Liang and his team, has solved the core issues of long development time, high costs, and poor combinatorial performance in traditional protein engineering through the synergistic closed-loop iteration of AI-powered "dry experiments" and efficient "wet experiments." This has provided dozens of high-performance protein products for fields such as in vitro diagnostics and synthetic biology.
Utilizing universal artificial intelligence for protein design has become a major trend in the field of protein engineering.
As we all know, proteins are the foundation of life systems and play an important role in cells, tissues, and organs. In addition to their biological significance, proteins are also crucial for a wide range of industrial applications and have extensive market value.
For example, in the biomedical field, they can serve as drug targets and therapeutic agents; in chemical engineering, they can act as key catalysts for various reactions.However, natural proteins in the environment typically require engineering modifications to enhance their activity, thermal stability, tolerance to extreme pH environments, and resistance to harsh solvents, among other indicators, before they can be applied in various industrial applications.
Traditional protein design necessitates a lengthy experimental research process that can span several years, not only consuming a significant amount of time and effort, but also increasingly failing to meet the transformation requirements for important proteins in many industrial applications.
In recent years, the development of deep learning technology has, to some extent, broken through the bottlenecks faced by traditional methods. The use of AI for the design and modification of proteins is gradually becoming a major trend in this field.
Independently developed general artificial intelligence for protein design, achieving precise protein prediction from sequence to function.According to the introduction, Hong Liang has many years of research experience in the field of AI protein design. He graduated from the Department of Physics at the University of Science and Technology of China for his undergraduate degree and from the Chinese University of Hong Kong for his master's degree. During his doctoral studies, he conducted research on the mechanisms of protein biophysics in the Department of Polymer Science at the University of Akron in the United States.
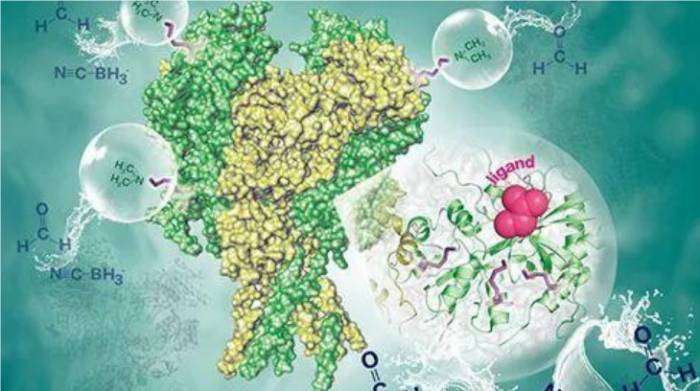
After completing his postdoctoral research at the Oak Ridge National Laboratory in the United States, he came to Shanghai Jiao Tong University and continued to study the performance of proteins by combining experimental and computational biology methods.
"In fact, these studies all belong to the category of 'post-interpretation'. In other words, it is about explaining some of the physical mechanisms of proteins, such as how their motion and various thermodynamic parameters affect their function," Hong Liang explained.
In 2020, the emergence of AlphaFold provided an opportunity for Hong Liang to start AI protein design research.
"Users only need to input the protein sequence into AlphaFold to get an accurate structural prediction, which is very shocking for the entire field of molecular biology."But AlphaFold only solved the problem from sequence to structure, not the problem from structure to function. We want to develop a general artificial intelligence that connects structure to function, completely breaking the shackles of traditional protein engineering methods," he said.
Therefore, he began to lead the team in AI protein design research and developed a general artificial intelligence for protein design based on pre-training in 2021, AccelProtein™ — unlike AlphaFold's prediction of structure, AccelProtein™ innovatively achieved precise protein design from sequence to function.
Specifically, the research group used pre-training methods to let AccelProtein™ learn all known protein sequences and structural features in nature, and explore and understand the mapping rules between protein sequences and functions in nature, thus developing a general model for AI protein design that can efficiently design proteins with good stability, high activity, and strong functionality.
So, how does this model achieve precise protein design?
According to Hong Liang, there are hundreds of millions of proteins with complete amino acid sequences known in nature, and the amino acid sequences of these proteins are arranged in a way that exists and is reasonable.After mastering these sequences, the team adopted a dual-task learning approach: on one hand, it helps the large model to grasp the language rules for protein sequence arrangement after pre-training learning; on the other hand, by constructing a database of protein labels at the billion level, it tags proteins to further improve the model's accuracy, thereby providing precise and efficient protein design, greatly reducing the cost of trial and error.
Compared with similar general artificial intelligence models, AccelProtein™ mainly has the following advantages.
Firstly, architectural advantage. The model architecture is simplified using geometric deep learning methods, which can reduce the model parameters while ensuring model accuracy, facilitating large-scale pre-training and inference.
Secondly, strategic advantage. By using small sample or even zero sample learning methods, the engineering generalization ability of the large model is improved, helping it to optimize protein performance with only a few wet experiment data, greatly improving the efficiency of protein design - projects that used to take 2-5 years to complete can now be completed in just 2-6 months with the support of AccelProtein™.
Thirdly, data advantage. Through cooperation with many domestic research institutions and enterprises, a rich and comprehensive high-precision protein sequence data has been obtained, especially some data under high temperature, low temperature or strong acid and strong alkali environments.In addition, this research group has also developed several other general AI protein models, achieving results comparable to those of similar achievements released by international teams such as Google and Meta.
According to the ProteinGym, a leaderboard for predicting the properties of protein mutations established by Harvard Medical School, the large model proposed by Hong Liang's team won the first place in non-retrieval methods and occupied half of the top ten seats in the overall ranking.
Among them, the large model for predicting eukaryotic proteins ranked first, the large model for predicting prokaryotic proteins ranked second, and the large model for predicting human proteins ranked third [2].
As mentioned above, in the entire protein design process, general artificial intelligence can empower protein modification without the need for or with only a small amount of wet experimental data. Does this mean that biological experiments no longer have a role to play?
Hong Liang holds a negative view on this.He believes that, firstly, AI still requires wet experiments to guide and adjust the direction when optimizing specific proteins.
Secondly, biologists can also propose more typical scientific questions through wet experiments, which facilitates the development of customized large models by the big model team based on these questions, thereby achieving batch protein design.
Founding an AI protein design company, more than ten protein products have been delivered
It is based on the achievements made in the field of AI protein design that Hong Liang founded Shanghai Tianyu Technology Co., Ltd. in 2021.The latter has already completed the delivery of results for more than ten protein design projects in less than three years and has obtained tens of millions of yuan in Pre-A round financing, with investment institutions including Yao Capital, Ganges River Capital, and others.
It is understood that the company's service scope has expanded to various industry fields such as innovative drugs, in vitro diagnostics, synthetic biology, etc.
At present and in the future, the research group is also trying to expand cooperation with more scientific research institutions and enterprises, hoping to set the best in the country and the best in the world in the field of protein engineering.
In Hong Liang's view, although China's biopharmaceutical industry already has strong strength, the profit ratio in the entire global product chain is still relatively low.
The reason is the lack of good ability to design upstream products, so that it cannot "break the situation" in a short time."After all, the design capabilities that international enterprises possess have been developed over the past century through a large amount of scientific research exploration and the accumulation of experimental data, as well as an incalculable accumulation of talent.
However, now with the universal artificial intelligence of proteins, we can bypass the development path of international enterprises and directly use AI to achieve 'changing lanes to overtake'.
Hong Liang said.
It can be imagined that once this road across the runway is opened, our country will be able to start a brand new competition with international enterprises in the field of synthetic biology and bio-pharmaceuticals."
Leave a Reply