Northeastern University in the United States proposes a video data enhancement m
Under the framework of deep learning, various data augmentation methods are widely used in the model training process due to their characteristic of alleviating overfitting, such as the rotation, scaling, and color change of images, etc.
However, Zhang Yitian, a third-year doctoral student at Northeastern University in the United States, and his team found that as an important attribute of image color, the variation of hue is overlooked in the current image recognition training framework.
There are two reasons for this:
Firstly, the changes in hue can lead to a significant color change in the appearance of the objects recognized in the image. For example, an image of a polar bear may look more like a brown bear after hue change, causing difficulties in model recognition.
Secondly, under the existing deep learning framework, the implementation of hue transformation is inefficient, leading to a very slow model training process.In a recent study, Zhang Yitian and colleagues re-examined the role of hue transformation in the modality of video data and observed a completely opposite phenomenon, that is, this operation can enhance the performance and generalization of video understanding models.
Advertisement
By further analyzing the reasons for the differences in performance under different modal data (images/videos), they found that for video understanding, the static appearance of the recognized object is not very important, and sometimes even detrimental.
For example, in a video where a person is holding a soccer ball and performing a basketball shooting action, if the model only understands the video based on the static appearance of the object, then the model may mistakenly believe that this person is playing soccer because it recognizes the soccer ball in the video.
Therefore, the team believes that in video data, it is more important to understand the temporal information conveyed by the video, such as understanding the shooting action itself, rather than understanding the appearance of the ball.
From this, it can be inferred the reason why hue transformation is effective in video understanding, that is, this operation can change the static appearance of the video data, allowing the model to learn representations that are invariant to static appearance, thereby implicitly encouraging the model to pay more attention to the temporal information in the video data.Even so, directly integrating the implementation of existing color tone transformations into the training process poses two issues:
Firstly, under the existing framework, the implementation of this operation is relatively inefficient, which can significantly slow down the training process.
Secondly, videos produced through color tone transformations exhibit noticeable distortions, leading to a shift in the distribution of the augmented training set compared to the actual data distribution, thereby limiting the model's performance.
To address the above issues, our research group has proposed a data augmentation method that enhances the model's ability to capture dynamic information in video data through reinforcement.
It consists of two parts:Part I refers to a method proposed by the team for achieving efficient hue transformation—SwapMix, which simulates hue variation by randomly shuffling the order of image channels and has achieved significant speed improvements on various platforms (CPU/GPU).
Part II refers to a universal method proposed by the research group to address the distribution shift caused by data augmentation—Variation Alignment.
This is done by constructing training pairs composed of regular samples and augmented samples, explicitly forcing the model to produce similar outputs, thereby preventing augmented samples from participating in the optimization process of cross-entropy loss, and allowing the model to learn representations based on invariant static appearances.
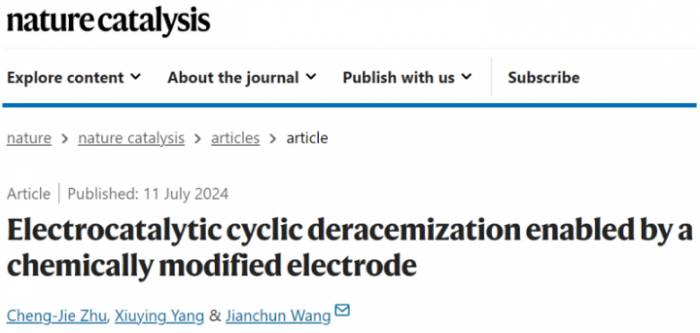
In general, they re-examined the role of hue transformation in video data, proving its effectiveness in video understanding.
At the same time, in response to many issues in traditional hue transformation implementation, the research group proposed a universal and simple solution, which can encourage the model to pay more attention to the dynamic information of the video, in order to better model the temporal information.In summary, this method has the following two advantages:
Firstly, the underlying idea of this method is very simple and universal, with no requirements or restrictions on the video understanding model itself, so it can be easily incorporated into the training of different models.
Secondly, since the operation of hue transformation has been overlooked in most previous work, this method can be well compatible with existing data augmentation methods and can achieve further performance improvement.
For example, for the current hot research on multi-modal large models, this method can be used in the training of video modality encoders, so that the model can extract better representations about video modalities.
In addition, the method proposed by the team to solve the distribution shift caused by hue transformation - Variation Alignment, is also a universal solution that can be used for different data augmentation methods.Specifically, existing data augmentation methods all have more or less distribution shift issues, and this method can be seen as a universal tool, so it can be used in different data augmentation methods to solve the negative impact brought by distribution shift and further enhance the performance and generalization ability of the model.
In fact, as early as his first year of doctoral studies, Zhang Yitian completed this work. At that time, he was very interested in video understanding.
Later, he found that the commonly used data augmentation methods in this field all came from the field of image recognition, and few people studied data augmentation methods specifically for video data.
So, he conceived the idea of exploring this direction, but at that time, he did not think very clearly about the research motivation and the reasons behind it.
Afterwards, he reorganized his thoughts and found that although the color transformation operation was deliberately ignored in the field of image recognition, it had very good effects in the field of video understanding.Subsequently, he conducted a more in-depth exploration in this small direction, creating the current method.
"Although completely abandoning our previous plan is equivalent to redoing the project, the final method is well-motivated and supported by strong research and analysis, so it was completed relatively smoothly," said Zhang Yitian.
In the end, the related paper was published in ICLR2024[1] with the title "Don't Judge by the Look: Towards Motion Coherent Video Representation."
Zhang Yitian served as the first author and corresponding author.
However, even though this method is a data augmentation method for video modality, the essence of the problem studied is still how to let the model learn a better representation of the video.This still has a certain difference from image recognition research, because Zhang Tian not only wants the model to understand the content of a single image, but also wants the model to understand the temporal information and changes in the video.
Therefore, in his subsequent research, he will explore how to use the reasoning ability of large language models to assist existing models in learning better representations about videos, thereby providing a better video encoder and constructing a more capable and multifunctional multimodal large model.
Leave a Reply