Scientists propose the GenAINet framework, making AI networks a comprehensive in
A few years ago, Professor Merouane Debbah, who was then the Chief Scientist at the AI and Digital Sciences Research Center of the Technology Innovation Institute (TII) in Abu Dhabi, led his team to successively launch the first Arabic large-scale model NOOR, as well as the Falcon series of large models (with scales of 7 billion parameters, 40 billion parameters, and 18 billion parameters).
The release of these major language models has also propelled the United Arab Emirates (UAE) to a leading position in the race for large models. Zou Hang, who is currently a researcher at the aforementioned unit, said, "This may be the first time that this young country, the UAE, has taken a leading position in international advanced technology."
The success of the Falcon series of large models has further strengthened the confidence and determination of the Technology Innovation Institute in Abu Dhabi, as well as the UAE government, to develop generative AI technologies, including large language models.
As a major force in researching AI-native networks, the Technology Innovation Institute in Abu Dhabi has applied AI technology in various aspects of communication systems, such as beam management, signal detection, separation, modulation, etc., and has achieved good results.
Advertisement
Paving the way for "AI + Communication"Currently, both the academic and industrial sectors of the communication field are paying great attention to generative AI technology.
In February 2024, at the Mobile World Congress held in Barcelona, Spain, several companies announced plans or products related to generative AI.
For example, Huawei released the first large model in the communication industry, and Qualcomm released the first communication chip supporting generative AI applications.
At the same time, AI-native networks are considered a key enabling technology for the next generation of mobile communication systems (6G), and foundational large models have the great potential to penetrate various vertical applications of the 6G network.
Research has found that with the increasing number of intelligent devices, future networks will not only be used for high-speed data transmission but also become an important part of intelligent decision-making.How to more efficiently deploy and apply generative AI technology in the wireless network environment, especially the deployment and application of large models that can understand and generate natural language (i.e., large language models), has become an essential question to answer in achieving "AI+ communication" applications.
However, integrating generative AI models into communication systems is not an easy task. A popular research approach in academia is to teach large language models to use various tools on the device, thereby expanding the application of large models in various fields.
These tools usually include internet information retrieval, calculators, local databases, various standard program interfaces, etc. By enhancing the ability of large models to use tools, intelligent agents based on large models can be obtained, and the large model is equivalent to the brain of the intelligent agent.
Only by endowing large models with the ability to use tools can the application scenarios of large models in communication be extensively expanded.
And only by allowing these intelligent agents not only to transmit data to each other but also to communicate and share knowledge can faster and better decisions be made. This is like allowing machines to exchange experiences and ideas, rather than just exchanging numbers and bits.The generative AI network framework GenAINet proposed by the Abu Dhabi Advanced Technology Research Institute team is precisely aimed at achieving this goal.
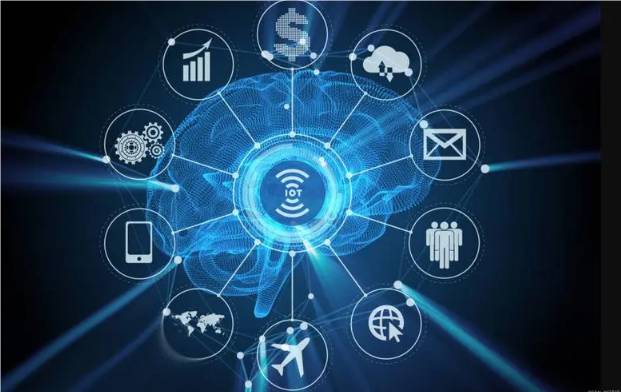
They hope that GenAINet will provide a new perspective and method for the application of generative AI in the field of communications, making future wireless networks not only faster and more widespread but also smarter and more efficient.
With the help of GenAINet, it is expected that in 6G networks, every connected device will become an intelligent agent driven by large models, such as base stations, personal computers, mobile phones, cars, robots, etc.
Utilizing the strong understanding, generation, and reasoning capabilities of large models, intelligent agents only need to transmit a small amount of semantic information to generate content, plan actions, and enhance knowledge. This new communication mode driven by knowledge will greatly enhance the application of vertical industries.
For example, in the vehicle network, autonomous driving cars can enhance road safety and driving efficiency by exchanging driving experiences they have learned in the past, such as obstacle prediction, braking prediction, and path planning capabilities.For instance, industrial robots in the Internet of Things collaborate with each other by exchanging semantic information, which enables them to accomplish complex tasks such as intelligent logistics, warehousing, and manufacturing.
In this way, a truly AI-native 6G network will be realized, where the network is no longer just a data transmission pipeline, but can become a vast integrated intelligence entity, thereby allowing operators to achieve greater commercial value in the wave of generative AI.
Addressing the Challenges of AI Application in 6G
The reason for conducting this study is that the team found the key issue for the large-scale application of AI in 6G is that traditional AI models struggle to achieve general effectiveness across diverse scenarios and tasks.To achieve the goal of AI-native networking, there is an urgent need for a network brain to connect various network devices and protocol stacks within the communication system. The multi-tasking and multi-scenario processing capabilities demonstrated by foundational large models in language are precisely in line with the objectives of the research group.
At the same time, there is a vast amount of data within communication networks, thus constructing a universal foundational large model for 6G is somewhat feasible.
The team believes that the intersection of large models and communication can generally be divided into two directions: large models empowering communication, and communication empowering large models.
Communication empowering large models involves utilizing or proposing new communication technologies to coordinate the deployment and services between multiple large models.
On the other hand, large models empowering communication involves using large models to solve a series of downstream issues in communication, such as resource optimization, protocol learning, and assisting in the generation of standards.In 2023, they began to experiment with "small" language models (such as GPT-2, the predecessor of the larger GPT-4 model) to classify technical texts of 3GPP (the global communication technology standard, 3rd Generation Partnership Project), and found the success rate to be quite high.
Later, they organized this preliminary result into a paper and published it at the flagship conference of the communication industry, GLOBECOM.
Subsequently, they started to try using models with larger parameter scales (including GPT4, Llama, and the Falcon series of large models developed by TII) to solve more practical communication problems.
In the research, how to construct a communication agent became the primary issue in front of them.
Thus, they explored the following issues in succession: how should large models use tools? How do large models plan complex communication tasks and break them down into simple executable tasks? And how do large models use local knowledge to enhance the feasibility of the final solution?After the research, the team believes that for large models to achieve large-scale application in the communication industry, it is essential to enable efficient communication of information between models with a huge number of parameters.
At the same time, what should be exchanged between large models is knowledge or experience that is highly conceptualized and abstracted, rather than merely transmitting raw information like traditional communication networks.
In other words, this method is more suitable for large models that can understand and complete instructions, which is of great significance for communication systems deeply integrated with AI technology.
In order to provide a practical solution for the industry, they conducted in-depth research on various large models and multi-agent application frameworks.
On this basis, they repeatedly experimented with the key ideas of generative AI networks and provided a brand-new end-to-end process framework.Later, they began to try larger-scale models, which brought them quite a few technical challenges. However, for researchers of large models, it is necessary to have a certain number of high-performance GPUs at hand.
Zou Hang said: "Fortunately, with the support of the leadership of the center, our computing resources are also increasing. In order to accelerate the research progress, we started from scratch to learn and master the technology of parameter compression and memory optimization of large models."
Finally, the relevant paper was submitted to IEEE Communications Magazine with the title "GenAINet: Enabling Wireless Collective Intelligence via Knowledge Transfer and Reasoning" and reviewed by industry experts.
Dr. Zou Hang, a researcher at the Abu Dhabi Advanced Technology Research Institute, Dr. Zhao Qiyang, the chief researcher, and Dr. Tian Yu, a researcher, are co-authors.
Generative AI is becoming more uncertain, and researchers need to take multiple paths.Interestingly, in the process of validating ideas, many debates surrounding the "future direction of generative AI" also constantly remind them to pay attention to different technological paths.
For large models, they require massive data training and resource training, but they cannot perceive environmental knowledge and abstract knowledge. Therefore, this method of "adding parameters" and "feeding data" is quite inefficient.
Meta has proposed a non-generative model architecture called JEPA, which has more efficient reasoning capabilities for complex tasks.
This aligns with the team's goal of building an efficient native AI network, so they plan to walk on two legs, that is, to use existing large model technology and explore new technological directions.
For people like Zou Hang, they believe that the development of large models must take the open-source path. Based on this, the team intends to further verify the application of this achievement in specific problems in the industry.It should be noted that all practical communication systems operate under the regulations of certain frameworks such as standards and protocols. Take the well-known 4G and 5G as examples; they are, in fact, a complex combination of a series of standards and protocols.
However, the knowledge of large models in the field of communication is relatively limited and currently only remains at the level of common sense.
In practice, the team found it difficult to make large models complete specific communication tasks according to the requirements of existing standards and protocols, such as network power optimization and network resource allocation.
Therefore, how to enhance the domain knowledge of large models in the communication industry will become the next hot topic of research in the communication industry and one of the subsequent tasks of the team.
In addition, as the cornerstone model of natural language processing, large models are naturally suitable for handling all tasks related to language. However, in the field of communication, a similar cornerstone model does not exist.The significant differences between electromagnetic signals, language signals, and visual signals make it challenging to construct a foundational model for communication.
The world model framework proposed by one of the "deep learning trinity," Yann LeCun, possesses a certain level of understanding and learning ability at an abstract level. Therefore, exploring the application of this framework in the field of communication is also a direction of great interest to the team.
However, the large-scale deployment and communication of large models will inevitably consume a large amount of communication and computational resources.
To enable multiple intelligent agents in the network to efficiently collaborate to complete complex tasks, it is necessary to allow large models to exchange only the most critical information to achieve the highest communication efficiency.
Thus, future intelligent agents will also need to possess functions such as perception, planning, decision-making, and memory. These will be the future research directions of the research group.
Leave a Reply